You checked into the hotel in Paris, and the whole week of vacation is ahead. A quiet breakfast is almost over, and it’s time to explore the city. Opening the map, you plan which sites you want to see and what streets to walk in between. So, the Eiffel Tower and Triumphal Arch are your landmarks and also points where you change routes. While Jacques-Chirac quay and Avenue Marceau are lines that connect your “space anchors.”
In computer vision, this technique is called landmark labeling or dot annotation, and it requires hiring a professional image or video labeler. In this article, you’ll find out when and why landmarking is used and how to hire a team of data annotators.
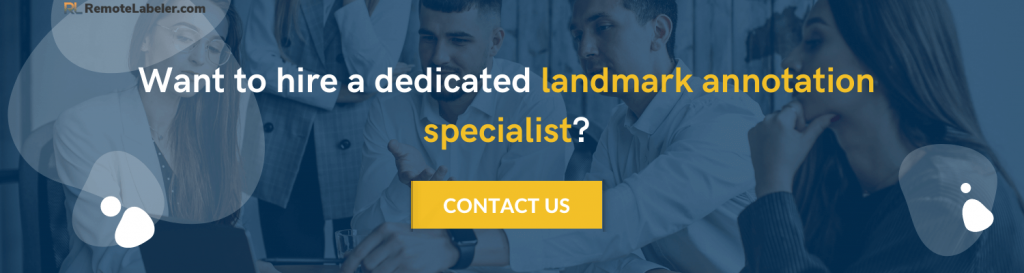
Overview of Landmark Annotation and Its Purposes
You probably know that all computer vision tools are trained by gigabytes of images and videos annotated by people. And there are several ways to let machines understand what is depicted on that digital media. People use bounding boxes, cuboids, lines, landmarking, polygonal, and semantic image segmentation techniques depending on project goals and object shapes.
You might have heard about setting landmarks because of facial landmark annotation. It helps detect and verify faces and gives face morphing and replacing more room. We enjoy these features in many entertaining apps. Some of them predict how we’ll look when we’re old; others can show what our children will look like. Some algorithms show our grand-grand parents smiling, and fantastic deepfakes make us feel excited and sometimes creepy.
The process of spotting landmarks isn’t complicated at first sight. Labelers do the key point annotation on a human face –– eyes, eyebrows, nose, lips, and shape its oval. And then facial recognition programs analyze distances between those points: mouth and nose, eyes, lips, chin, etc. These sizes make each face unique, and by them, programs can identify people. However, for making correct body landmarks machine learning projects also require labelers to spot more and less substantial points and join them.
Landmarking: Use Cases
The landmark approach is highly effective for creating a facial recognition dataset, and by 2025 its world market revenue will reach $8.5 million. And here also are two other applications of this data annotation method:
- Facial gesture recognition. Understanding human face impressions is possible due to data annotation and the FACS (Facial Action Coding Systems). These systems were worked out in 1970 and updated in 2002, but they still help identify mood and sentiment. This document describes so-called Action Units that engage various face muscles like “Lip Corner Puller” or “Cheek Raiser.” The combination of these two Units, for example, represents happiness.
- Human pose estimation (HSE). Main points on a human body connected in pairs form a 2D or 3D skeleton, assessing human pose. Such skeletons are used in fitness apps to check if a user exercises correctly. Cameras with computer vision’s posture measure can detect and help avoid incidents in production halls or construction areas.
- Counting similar small objects from the bird-eye view. This application isn’t as widely used as the previous two. Still, putting one dot per object seems to serve counting purposes ideally. Labelers can help spot various objects on an image taken by drones. Labelers can mark cars in parking, trees, and ponds in parks, houses, etc. –– every small item on aerial views of cities.
Industries Where Landmark Detection Is Used
Since the landmarking approach allows detecting and emulating body movements and identifying faces and emotions, the spheres of its application are numerous. For example:
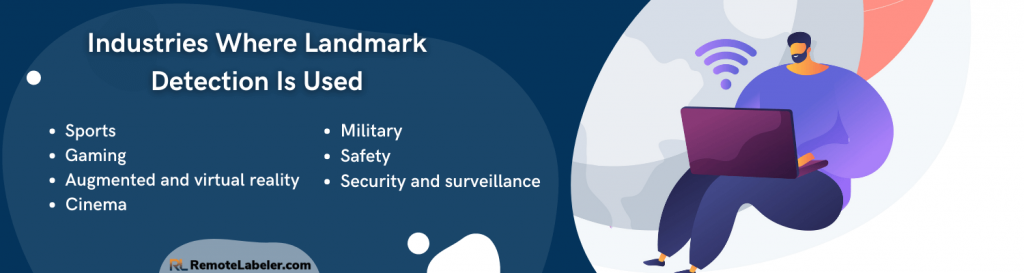
- Sports. Multiple fitness apps use this technology to assess how well users repeat movements and poses.
- Gaming. Almost every game uses landmark annotation to simulate human-like movements.
- Deepfakes. Together with face replacement and morphing, deepfakes are really a breakthrough in technology.
- Augmented and virtual reality. Like in gaming, landmark tagging helps create realistic characters and naturally-moving creatures.
- Cinema. Amazing creatures move so naturally because they learn from humans, animals, birds, and insects.
- Military. Drones can detect or monitor weapon reserves and observe army forces’ activities by spotting landmarks.
- Safety. Monitoring crosswalks to detect human figures can be used, for example, to make self-driving cars safer.
- Security and surveillance. Cameras equipped with computer vision can signal any unusual human activity or changing conditions.
Our list of Artificial Intelligence spheres where people use landmark labeling isn’t full as it constantly grows. Humans teach machines to assist them in healthcare, agriculture, robotics, and other industries.
How to Label Landmarks Correctly: Method Challenges
Before starting the annotation of facial landmarks or key points on human bodies, data labelers read project guidelines and agree on the software to use. This will help get the most accurate results; however, limitations still exist:
- The limited volume of model training datasets. If you want your project to succeed, the training dataset for your ML model should include images from different angles. Recording video from various perspectives also can teach algorithms to estimate a 3D pose of a person better. But having several cameras and processing those videos requires more computing resources and, thus, more investment.
- Improper background and illumination. This is a common problem for all data annotation methods as they mainly process real-life photos and videos. Too bright or too shadowed pictures, especially if the background is specky make it harder to label body landmarks. Other people and objects on the image, appearing obstacles, and uncommon poses also complicate landmark identification and affect the recognition rate.
- Hidden object parts. The algorithms can’t learn appropriately if input data contains many pictures with hidden body parts. Bending, twisting, turning, slanting back –– all of these body postures make the work of landmark markers harder. And ML models can get confused by inaccurate, improper, or incomplete landmark settings. This will result in poor recognition of human movements, gestures, emotions.
Our Landmark Annotation Teams Staffing Services
We’re glad to deliver top-notch outsourcing services and equip your business with first-class landmark annotators. And working with us offers clients several benefits.
Benefits of Landmarking with Our Teams
- Reduced costs. Since we work with data annotators from Ukraine, you have a chance to save on their payrolls. That’s because average salaries in Ukraine are significantly lower than in Western Europe and the USA. So, outsourcing remote teams with us is cost-effective.
- Experienced and skilled staff. Ukraine boasts well-trained labelers with deep knowledge on the topic and impressive experience. Contracting us, you get access to an excellent talent pool and can equip your AI project with business-focused professionals in several weeks.
- One amount to settle. You’ll forget about monthly payroll payments, transferring rental fees with utilities, or any other administrative settlements. We offer a fixed fee payable every month and let you focus on work tasks. And, of course, you get a breakdown of all expenses that are always pre-agreed.
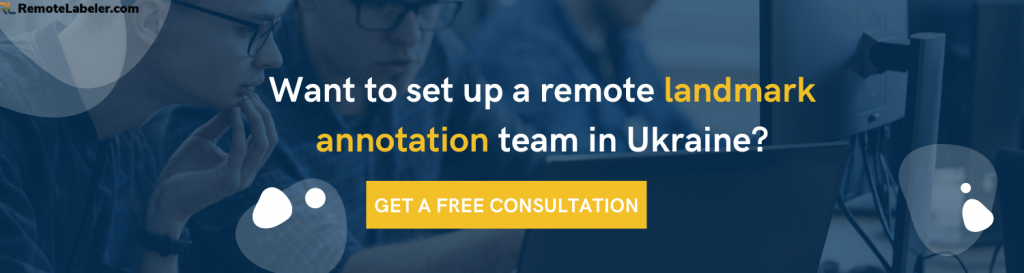
How We Assemble Landmark Annotation Teams
Our cooperation is always client-oriented and flexible, so you get your team ready for work within the shortest period. And that’s how we do it:
- You complete the contact form below, and within a couple of hours, our managers will call you. We need to determine all the requirements for your future team and agree on the job description.
- We post the vacancy on local job portals and start sourcing candidates. We arrange interviews and already have the first candidates in 2-3 weeks to show you and our recommendations/notes on each.
- We send you a few CVs for each position and wait until you communicate the candidates, test, or interview your potential human posture recognition computer vision team. And, as soon as you choose the ones you’ll work with, we send them preliminarily agreed job offers.
- We sign a cooperation agreement, issue an invoice and send it to you for settlement. From now on, we curate your teammates in all administrative and HR issues, and you think of work only.
Hire a high-quality landmark annotation team and launch your AI project!
- Emerging Trends and Future Outlook: The Data Labeling Industry in 2024-2030 - December 8, 2023
- Landmark Annotation: Key Points - November 6, 2023
- All You Should Know About Bounding Box Annotation - November 5, 2023