You sit in a chaise-longue with a cocktail –– being totally free for the next week. This sun, ocean, and incredible view stop the time for a while, and you feel happy. You can see the line where the sky meets water and watch the little clouds go by –– not a single detail of this “snapshot” in your mind is missed. Well, we readily distinguish things and phenomena around us, but computers need to have every category separated or segmented to “see” the same.
In this article, you’ll learn what semantic segmentation is, how it helps computer vision projects and why we can be your image annotation outsourcing provider.
What Semantic Image Segmentation Is
Semantic segmentation splits an image into segments (classes), not leaving a single pixel unattributed. In our example from the Maldives above, there are three segments: the sun, the ocean, and the sky. Labelers use different colors to match each, especially minding the borders. This way, every single pixel belongs to a class and has its color. So, this technique is called semantic pixel-wise image labeling.
If we, for example, needed to mark clouds as the fourth class, we could do this in two ways. We’d label all five clouds in one color following the semantic segmentation approach. But there’s an even more detailed way –– to “sub-label” each as a separate object within a class. So, each cloud would belong to the same class but represent a particular object. This method is called instance segmentation.
Semantic image segmentation is a detailed object localization on an image –– in contrast to a more general bounding boxes approach. That’s because of the image thresholding, which helps decompose the scene on it. This allows separating, moving, or deleting any of the chosen classes offering plenty of opportunities. That’s what semantic segmentation offers, starting from a creative photo and video edition and ending with autonomous cars.
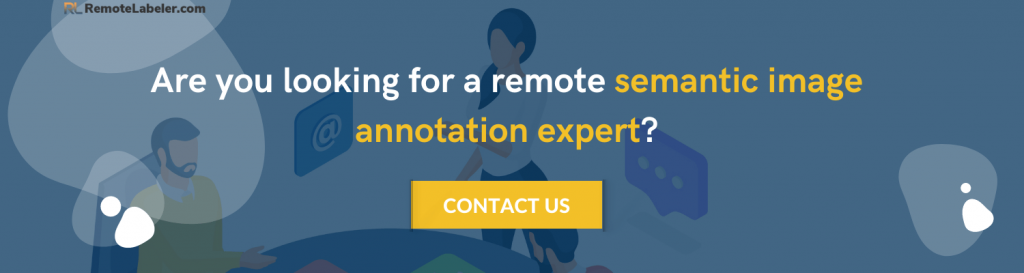
Types and Modes of Segmentation Image Processing
There are two opposite approaches to image segmentation: the first considers pixel similarities, and the second takes into account their differences. Professional labelers choose between them, minding image properties and project goals.
- Region-based method (looking for similarities). This approach allows growing regions from a seed pixel. Annotators connect each next pixel if it’s similar to the main one, and thus, the segmentation area extends. They use specific predefined similarity criteria to assess each case. So, in general, you choose one pixel and grow its area by adding similar ones.
- Edges-based method (looking for differences). In this method, labelers need to find pixels representing the object’s edges. If pixels have vectors of the same direction, they should be united. This method works best if the background is in high contrast to the object. But it’s less effective when color transitions are smooth. So, this approach separates edges from the object itself.
There are other methods that use image segmentation deep learning algorithms, including AI-based neural networks and different programming approaches.
Importance of Object Segmentation and How It Works
Object identification can save human lives, and that’s why accurate semantic segmentation is crucial. Here are only several examples of how AI algorithms can help people avoid danger or get timely assistance in case of injury.
- Detecting cancer. Standard screening methods don’t always detect cancer signs, especially at an early stage. Though cancer cells have another shape, sometimes they still remain unnoticeable to the human eye. But adequately trained AI algorithms can be much more accurate in cancer identification. However, the greatest challenge of such projects is the lack of properly trained datasets.
- Monitoring construction sites and production areas. The possibility to monitor how workers move (through the safe paths marked on the floor), if they use required safety accessories, or leave machines unattended can help avoid severe injuries and sometimes save lives. Though managers always keep safety standards high, workers sometimes neglect them.
- Autonomous vehicles. More cars are manufactured autonomous-ready. This means that AI-based software supporting this function has to be perfectly trained. The ML models not only have to detect and identify objects they meet on the road or around the car. The main challenge is to be able to respond to this information immediately. So, real-time monitoring algorithms have to be predictive to keep passengers away from dangerous situations.
Semantic Image Segmentation with Deep Learning: Major Services
AI projects trained with semantic image segmentation datasets have many applications in our life.
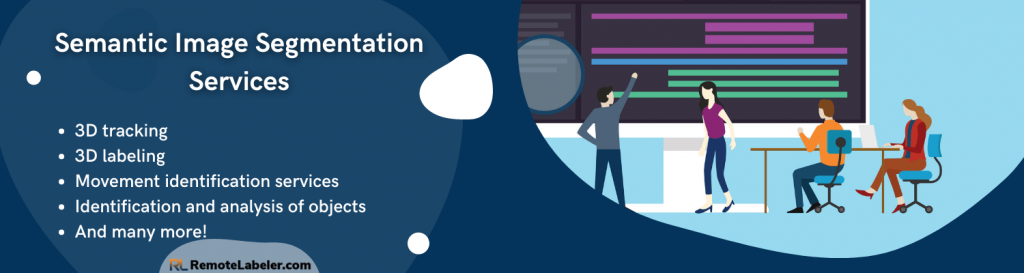
And here are the primary services that they help deliver:
- 3D tracking. Software that can analyze objects moving in 3D space is widespread. These programs serve multiple purposes like security surveillance, augmented reality, robotics, automotive.
- 3D labeling. Semantic segmentation can automate the classification of big datasets. This solution works best to distinguish various object classes and separate items belonging to that class.
- Movement identification services. Fitness apps that help users train from home analyze movements using a semantic segmentation approach. This category also includes systems that offer interactive communication or devices within IoT infrastructures.
- Identification and analysis of objects. This service is top-rated across all industries using computer vision and deep learning. So, multiple AI-based projects use datasets processed with semantic segmentation.
- Others. Use cases of Machine Learning models that learn from training datasets are numerous. That’s why the number of services provided by object identification algorithms will also grow.
Applications of Pixel Level Image Segmentation
Artificial Intelligence projects use semantic image segmentation for various purposes.
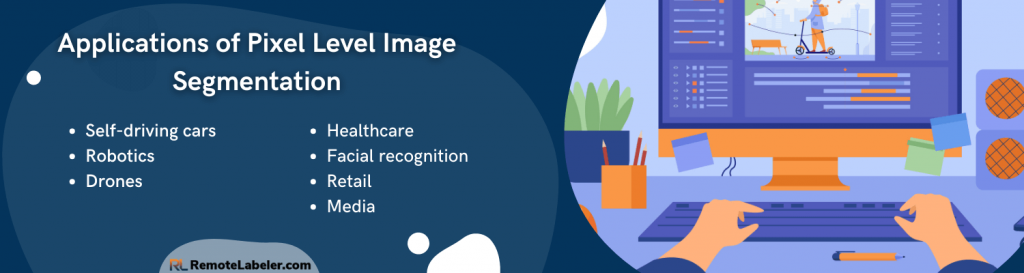
And here are only some applications of this technology:
- Self-driving cars. Semantic segmentation can help autonomous vehicles effectively detect pedestrians, cars, and other objects on the road. And algorithms trained with this method enhanced with polyline annotation can ensure a successful driving experience.
- Robotics. In agriculture, robots can identify fruits and vegetables ready for harvesting, understand if there’s any disease that needs to be treated, do pest control, etc.
- Drones. Aerial-view images made by drones help people see farm fields or military objects. And here, semantic segmentation can show the location of crucial objects, check stocks, and monitor plant growth.
- Healthcare. Medical image segmentation detects abnormal areas on MRI and CT scans. This way, segmentation technology helps doctors make diagnoses faster and with minimal errors. This means more patients can get proper and timely treatment.
- Facial recognition. Image segmentation face detection allows detecting parts on a human face (eyes, lips, nose, skin, hair, etc.). Then algorithms can identify facial expressions, age, ethnic origin, and other parameters of an individual.
- Retail. Clothing categorization helps marketers and merchandisers test various strategies. And for the security department, image segmentation helps identify products that people take without payment.
- Media. Image and video processing software and applications use semantic segmentation for cutting, replacing, and adding objects and process files of various formats.
This list isn’t complete as the spheres that use AI algorithms trained by image segmentation datasets only grow. IoT, manufacturing, geospatial technology, smart parking are only some to add.
Reasons to Outsource Semantic Segmentation
Semantic segmentation is a complex process where each pixel of an image is classified into a specific entity or class. For example, if image semantic segmentation deals with a picture of a cat on a couch, each pixel is assigned to such classes as “cat” and “couch” as well as any other predetermined classes. The more complex the image is, the more detailed pixel annotation could be. For instance, people can be identified as “humans” or separated into “female” and “male” categories.
It is a time-consuming process that requires a specific skill set, qualifications, and attention to detail. Semantic images are commonly used in various ML and AI systems such as autonomous systems for vehicles, medical diagnostic algorithms, or geographical data analysis solutions.
There are several reasons why outsourcing semantic segmentation services are widely popular. The main ones are:
- Time efficiency. Working with a professional provider means that you can get a properly annotated set of data quickly. It is a labor-intensive process that usually cannot be done with only internal resources. But if you delegate it to specialists, your in-house team gets to focus on the core of their responsibilities.
- Cost saving. It is one of the major reasons why outsourcing is beneficial for many businesses. By working with a remote team, a company can save a lot of money on the hiring process, buying hardware and software, and training new staff.
- High-quality data. Semantic segmentation images require a particular approach and toolset to be done correctly and without any errors. Small inconsistencies and mistakes done because of the lack of experience or standardized procedures might result in time waste and money loss. Specialists, on the contrary, know exactly how to approach the task and provide excellent results in the shortest period possible.
- Avoid internal bias. Delegating the semantic segmentation to a third party allows for avoiding any type of internal bias that might be present. You access an objective perspective that is incredibly important to Machine Learning projects. If the bias is present, the solution might suffer in terms of flexibility, functionality, and versatility. Professionals minimize such risks by incorporating proper procedures, and protocols, and minimizing sample bias.
- Scalability. If you are working on such a project for the first time it might be challenging to establish precisely the amount of data you would need. Outsourcing ensures easy scalability whether you need to scale up or down. So if the set of information for segmentation grows rapidly, you still can get the same results on time. Working with a large amount of information requires decent manpower that the majority of businesses do not have internally. Outsourcing allows for solving this issue cost-effectively and efficiently.
- Versatile and trained talent. Another huge reason to consider outsourcing is that it gives a company access to a wider talent pool. This means more versatility and opportunity to find the exact skill set you need for your specific goals and purposes. Trained specialists can tackle the most complex tasks with ease and on time.
Outsourcing a Machine Learning Image Segmentation Team: Why We
If you have a ready dataset for image segmentation but still haven’t found a team of data labelers, then consider the benefits we offer.
- You can spend less on monthly salaries. We’re glad to work with Ukrainian image segmentation specialists and offer cost-effective solutions to our clients. That’s because the average payroll of a Ukraine-based data labeler is significantly lower than his or her colleague from the USA and Western Europe. So, with us, you can save up to 15% of your costs.
- You pay for what you get. Our services are very flexible and client-oriented. For you, this principle is translated into another opportunity to optimize costs. We can assemble a computer vision segmentation team of as many professionals as you need. But if with the time you need to grow it or put your AI project on hold for a while, we remain flexible.
- You can get top professionals aboard. The outsourcing model offers exceptional opportunities for hiring annotators with extensive experience and profound industry-relevant skills. This way, you have a chance to make your Machine Learning project stand out with high-level data labelers –– and with minimal investment.
- You can focus on business tasks. Our staffing model assumes that we take on all administrative issues related to your team. This means that you can stay focused exclusively on work-related tasks, and we will arrange working infrastructure, ensure employee retention and do all the paperwork.
- You pay only one amount. Every month, we issue a single invoice, so only one fixed fee covers the expenses of your segmentation computer vision team. You don’t have to settle various payments like payrolls, taxes, rentals, utilities. Doing onboarding new members and arranging team building are also our tasks.
It takes us 2-4 weeks to send you the CVs of the candidates who ideally match your job description and requirements. And all you need to do is send us a contact form below.
Learn how to equip your business with a team of semantic image segmentation professionals!
- Emerging Trends and Future Outlook: The Data Labeling Industry in 2024-2030 - December 8, 2023
- Landmark Annotation: Key Points - November 6, 2023
- All You Should Know About Bounding Box Annotation - November 5, 2023