In the modern era of artificial intelligence (AI) and machine learning robotics, an abundance of fields, from surveillance to sports performance analysis, significantly benefit from person tracking. With the prolific expansion of such technology, the ability to accurately track individuals becomes increasingly critical. The process of person tracking involves coding, labeling, and structuring data, ensuring its readiness for advanced algorithmic interpretation. This necessitates a deep dive into the realm of object annotation and how its advancement can dramatically boost person tracking accuracy.
Understanding Basic Object Annotation and Its Limitations
Object annotation, at its most basic level, is the process of labeling objects within images or video frames to enable object detection by machine learning algorithms. It operates as the cornerstone of object tracking in computer vision, imbuing AI systems with the ability to recognize and differentiate various objects within their field of view. However, the traditional object annotation techniques come with their fair share of limitations:
- Limited Accuracy: Basic bounding box annotations often fail to capture the precise shape and orientation of the objects, leading to less accurate detection and tracking.
- Inadequate for Complex Scenes: Basic annotation techniques might prove insufficient in dealing with complex, cluttered scenes where multiple objects overlap.
- Manual Intervention: The need for human intervention in the annotation process can result in errors, inconsistencies, and increased time consumption.
The Importance of Accuracy in Person Tracking
Person tracking operates on a dual-axis of challenge and opportunity. Its criticality stems from the fact that it serves as a lynchpin for several operations. Any inaccuracies can lead to a ripple effect of inefficiencies, potentially creating grave repercussions.
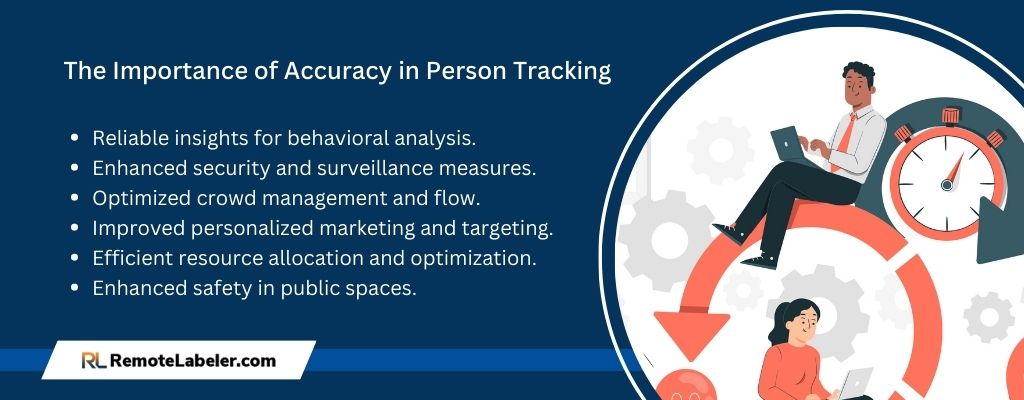
Promoting Security and Surveillance
In the realm of security and surveillance, person tracking is a pivotal component. Accurate tracking can aid in recognizing suspicious behavior, preventing potential threats, and enabling swift actions. An incorrect identification, on the other hand, can trigger false alarms and squander resources.
Enabling Autonomous Vehicles and Drones
For autonomous vehicles and drones, precise person tracking is essential to ensure safety. These systems must accurately identify and track people in their vicinity to avoid accidents and navigate effectively.
Empowering Healthcare Monitoring
In healthcare, person tracking has the potential to revolutionize patient monitoring, particularly for elderly or differently-abled individuals. It can help identify unusual behaviors or movements that may indicate a health concern. However, inaccuracies could lead to false alarms or, worse, missed critical signals.
Enhancing Retail Experience
In retail, person tracking can help understand customer behavior, thereby enabling personalized experiences. If inaccuracies creep into the tracking system, it can lead to misguided strategies and lost opportunities.
Through meticulous object annotation, person tracking accuracy can be significantly enhanced. By amalgamating advanced annotation techniques with cutting-edge algorithms, we can track objects, specifically people, with higher precision, advancing numerous fields with the power of technology. Object tracking, once a figment of science fiction, is now an accessible reality, changing the way we interact with our surroundings.
Enhancing User Experience in Augmented Reality (AR)
Augmented Reality (AR) platforms heavily rely on accurate person tracking for immersive and realistic user experiences. Without pinpoint accuracy in object tracking, the AR systems may fail to convincingly incorporate the user’s image into the simulated environment.
Boosting Performance in Sports Analysis
In sports analysis, the difference between a winning and losing strategy often boils down to granular details. Accurate person tracking can help sports teams to dissect and understand the gameplay more precisely, leading to better-informed strategies and improved performance.
Advanced Object Annotation Techniques for Person and Object Tracking
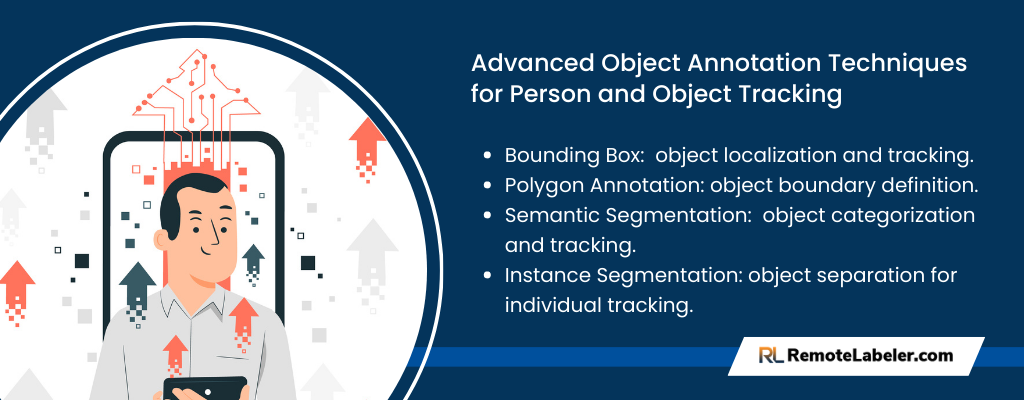
Understanding the diverse techniques of object annotation and their relevance to both person and object tracking deep learning can guide us towards the right methodologies for improving tracking accuracy.
Bounding Box Annotation
Bounding box annotation demarcates the object of interest within a rectangular box. This methodology provides a general area of the object’s presence in the frame and is advantageous for its simplicity and efficiency. Bounding box annotation is often utilized in video object tracking and object tracking and detection, where the objective is to identify the location and movement of objects.
Polygon Annotation
Polygon annotation takes precision a step further. By forming a polygon around an object, it captures irregularly shaped entities more accurately than a bounding box. This technique is typically used when the object of interest has a complex shape or the task requires a higher level of detail, often critical in visual object tracking.
Semantic Segmentation
Semantic segmentation assigns a class label to every pixel in the image, resulting in a comprehensive, pixel-wise map of the image. This approach is particularly useful in applications where context and detail are vital, such as autonomous vehicle navigation and precision agriculture.
Instance Segmentation
Instance segmentation not only classifies each pixel like semantic segmentation but also differentiates between separate objects of the same class. This sophisticated technique proves to be invaluable in scenarios that necessitate the identification of individual objects, a critical aspect of deep learning tracking.
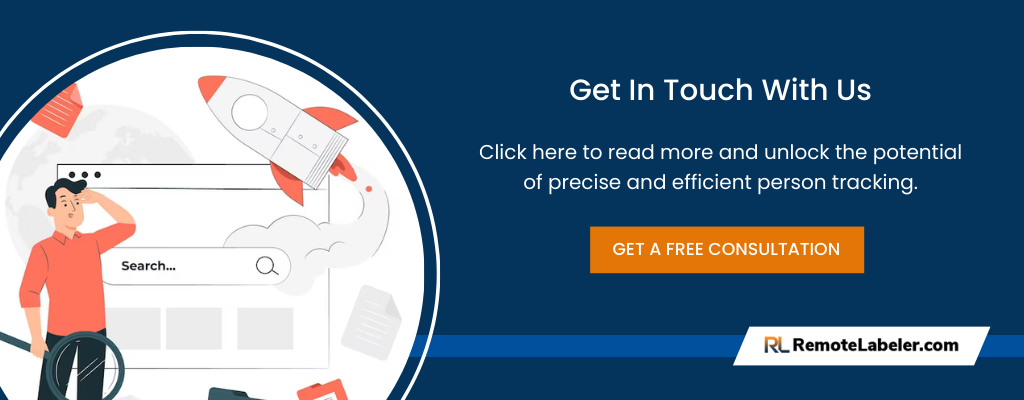
Professional Outsourcing Services: The Remote Labeler Advantage
Implementing these advanced object annotation techniques can be resource-intensive. Professional outsourcing services such as Remote Labeler offer a seamless solution, providing top-notch object detection and annotation services. With a keen focus on quality, precision, and speed, Remote Labeler uses state-of-the-art annotation tools and a human tracking dataset to train deep learning models effectively.
Partnering with Remote Labeler offers several benefits:
- Accuracy: With rigorous quality control processes, Remote Labeler ensures high-quality, accurate annotations.
- Scalability: As your needs grow, Remote Labeler can swiftly scale up operations, providing consistent and timely delivery.
- Security: Remote Labeler understands the value of your data and prioritizes maintaining its confidentiality and security.
- Expertise: With a wealth of experience, Remote Labeler brings expertise in advanced annotation techniques, enhancing the value they deliver.
Conclusion
Advanced object annotation techniques are a cornerstone in the journey towards achieving accurate person tracking. Whether through bounding box annotation, polygon annotation, semantic segmentation, or instance segmentation, each technique contributes significantly to the effectiveness of object tracking deep learning. By understanding the nuances of these methodologies, businesses can better select and apply the right techniques to their unique needs. Professional outsourcing services like Remote Labeler can offer an efficient way to incorporate these methodologies, providing quality, security, scalability, and expertise.
As we venture further into the era of artificial intelligence, the relevance and impact of these techniques will only continue to grow. Leveraging them effectively is key to unlocking the potential of deep learning human tracking, thereby fueling advancements across numerous fields.
Get in touch with Remote Labeler today and discover the difference with advanced annotation techniques.
- Emerging Trends and Future Outlook: The Data Labeling Industry in 2024-2030 - December 8, 2023
- Landmark Annotation: Key Points - November 6, 2023
- All You Should Know About Bounding Box Annotation - November 5, 2023