The advent of biomedical image analysis has revolutionized the landscape of medical research and healthcare. Leveraging advanced imaging technologies, this realm is burgeoning with potential and has kindled significant innovations in medical diagnostics, clinical practices, and biomedical research. The ability to scrutinize intricate biological processes at an unprecedented level has opened new horizons of understanding. These insights can shape our comprehension of diseases, contribute to early diagnosis, and guide the development of novel therapeutics.
Notwithstanding, the extraction of these insights is contingent on the interpretation and analysis of colossal volumes of biomedical imagery. This critical juncture is where data annotation companies play an indispensable role. Through meticulous annotation and labeling of biomedical images, they render structure and coherence to the complex data. As a conduit for machine learning and AI applications, their work paves the way for next-generation breakthroughs in medical research and patient care.
Understanding Biomedical Image Analysis
Biomedical image analysis, in essence, refers to the process of converting visual information into quantitative data. It forms the linchpin of many branches of medicine and biology, aiding in the diagnosis, treatment, and study of numerous conditions. This discipline has transformed the way we investigate and comprehend complex biological phenomena, propelling us into a new era of digital medicine.
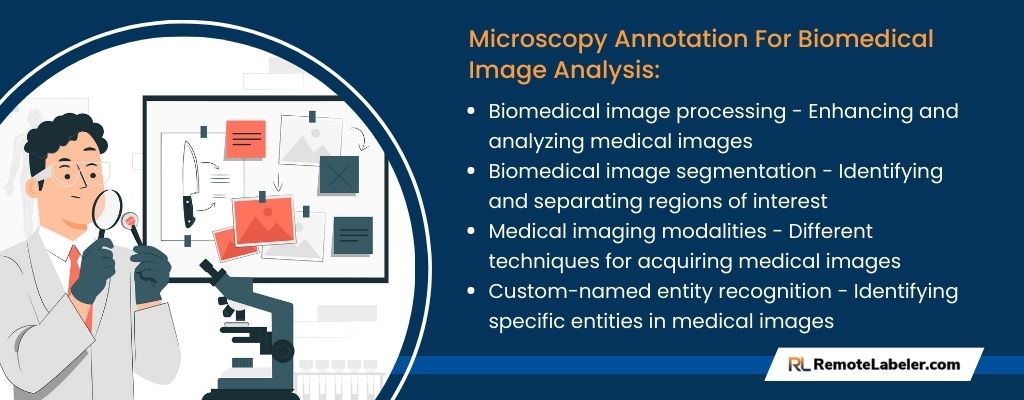
- Medical imaging modalities: Biomedical image analysis makes use of a spectrum of imaging modalities, such as Magnetic Resonance Imaging (MRI), Computed Tomography (CT), Positron Emission Tomography (PET), and microscopy. Each of these modalities holds its own unique set of challenges and opportunities, enabling different perspectives into the human body and cellular processes.
- Biomedical image processing: This phase involves the manipulation of images to enhance their quality or to extract meaningful features. A multitude of techniques for biomedical image processing exists, encompassing filtering, smoothing, edge detection, and noise reduction, to name a few.
- Biomedical image segmentation: Image segmentation holds a pivotal role in image analysis, involving the division of an image into multiple segments or ‘regions of interest’. These regions can then be studied individually for their unique properties such as shape, texture, and color, granting a detailed insight into the subject of study.
- Custom named entity recognition: This capability refers to the ability of AI systems to identify and classify elements in text data. In the context of biomedical image analysis, named entity recognition could be utilized to identify and categorize elements in annotations or metadata associated with images.
The profundity of biomedical image analysis extends beyond mere visual examination. It marries multiple scientific disciplines and technologies, bringing together facets of computer science, mathematics, physics, and biology. Each step in the process, from the choice of imaging modality to the processing and segmentation of the images, is a critical component that builds towards a comprehensive understanding of the biological system under study. With the advent of AI and machine learning, the possibilities for this field are boundless and replete with opportunities for innovation.
The Power of AI in Biomedical Image Analysis
Harnessing artificial intelligence for biomedical image analysis constitutes a paradigm shift in healthcare and medical research. Given the extensive and diverse nature of biomedical image data, manual analysis is a Herculean task, fraught with inconsistencies and inefficiencies. Conversely, AI, with its capacity to process vast data volumes at lightning speed and detect intricate patterns, is poised to transfigure this landscape.
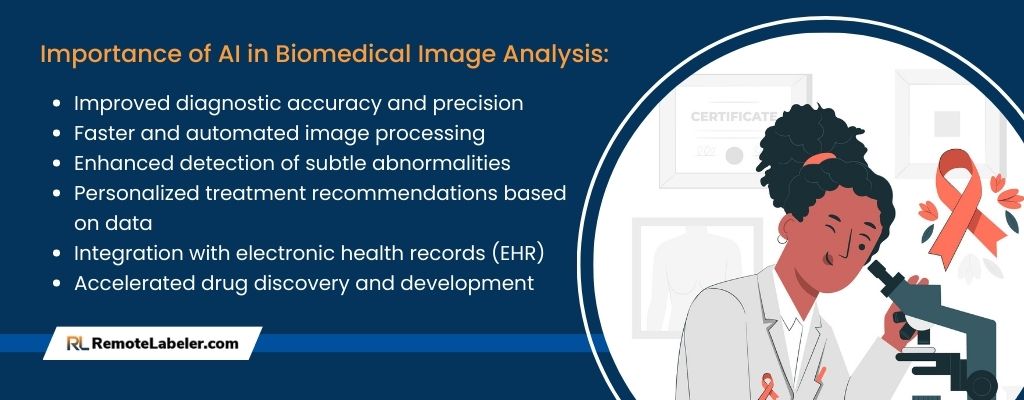
Enhanced Efficiency
The transformative power of AI in biomedical image analysis is most evident in its ability to augment efficiency. Traditional image analysis methods, albeit accurate, are labor-intensive and time-consuming, making them unsuitable for scenarios requiring rapid diagnostics. AI algorithms, through automated image analysis, can significantly truncate the time taken to analyze extensive data volumes. This increased speed is particularly advantageous in the biomedical field, where expedited diagnosis and intervention can have profound implications on patient outcomes.
Improved Accuracy
Accuracy forms the bedrock of any medical procedure, and it is here that AI outshines human capabilities. AI algorithms, trained on meticulously annotated biomedical image data, can analyze images with exceptional precision. This accuracy is particularly salient in tasks such as biomedical image segmentation, a process that requires high-level expertise and attention to detail. An AI’s potential to recognize intricate patterns and draw inferences is beyond human capabilities, resulting in more precise and consistent results, which in turn translates to accurate diagnoses and effective treatment strategies.
Predictive Analytics and Proactive Healthcare
In addition to efficiency and accuracy, AI takes biomedical image analysis a notch higher by empowering predictive analytics. By analyzing historical and real-time data, AI models can predict disease progression, patient response to treatments, and potential health risks. This predictive ability facilitates proactive healthcare, enabling medical professionals to anticipate complications and intervene early. It underscores the significance of AI in shaping a future where preventive care supersedes reactive approaches, making healthcare more efficient and patient-centric.
Applications of Microscopy Data Annotation
Microscopy data annotation plays a pivotal role across a multitude of applications, from clinical diagnostics to advanced biomedical research. It serves as the foundation for the implementation of AI in various biomedical imaging modalities, thereby fueling cutting-edge discoveries and innovations.
- Clinical Diagnostics: Microscopy data annotation enables AI models to accurately identify and quantify biological entities in microscopy images, such as cells or bacteria. This is particularly crucial in fields like pathology and microbiology where precise detection and enumeration of these entities can guide accurate diagnoses and treatment strategies.
- Biomedical Research: In the realm of biomedical research, data annotation can facilitate the understanding of complex biological processes. Annotated images enable AI models to track cellular behavior over time or under different experimental conditions, leading to new insights and research breakthroughs.
- Drug Discovery: In the pharmaceutical industry, microscopy data annotation can contribute to the discovery and development of new drugs. By facilitating the analysis of cellular responses to various drug compounds, it can aid in determining the effectiveness of potential therapeutic candidates.
- Precision Medicine: Microscopy data annotation can also contribute to the growth of precision medicine. By training AI models to recognize patterns associated with specific diseases, treatments can be tailored to the individual patient, thereby improving efficacy and reducing side-effects.
The role of microscopy data annotation in these applications underlines its importance in the progression of healthcare and medical research. The value it adds is immeasurable, as it makes possible the complex task of deciphering vast amounts of biomedical imagery, thus empowering AI to revolutionize medical practice and research.
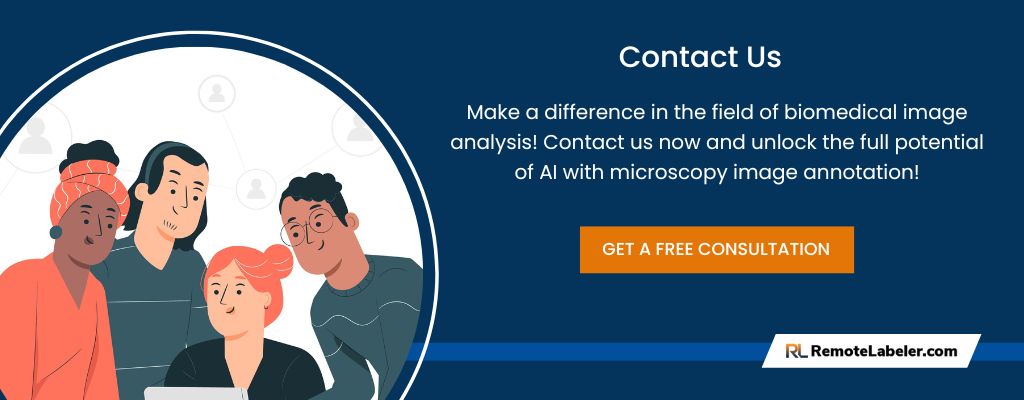
Challenges and Best Practices in Microscopy Data Annotation
Microscopy data annotation, a fundamental pillar for training AI in biomedical image analysis, often encounters several challenges that can stymie the advancement of AI models. These challenges range from the technical aspects of annotation to maintaining consistency and quality across vast datasets.
- Complexity and diversity of data: Biomedical images, particularly those derived from microscopy, often contain highly complex and diverse data. The task of accurately identifying and annotating these elements can be daunting and time-consuming.
- Quality and consistency: The reliability of AI models is directly proportional to the quality and consistency of the annotated data. Any inaccuracies in annotation can lead to erroneous AI predictions, impacting patient diagnosis and treatment.
- Adapting to evolving scientific knowledge: The field of biomedicine is continually evolving. The annotations must accurately reflect the current state of knowledge, requiring constant updates and revisions.
While these challenges may seem formidable, companies like Remote Labeler are well-equipped to handle them. As a trusted data annotation provider, Remote Labeler brings to the table a robust suite of solutions to overcome these challenges:
- Expert Annotators: Remote Labeler employs a team of expert annotators who possess deep domain knowledge, enabling them to navigate the complexity and diversity of biomedical images effectively.
- Rigorous Quality Assurance: Through stringent quality checks and protocols, Remote Labeler ensures that the quality and consistency of annotations are maintained, thereby enhancing the reliability of AI models.
- Dynamic Updates: Leveraging state-of-the-art tools, Remote Labeler stays abreast of the latest scientific developments. Annotations are dynamically updated to reflect current knowledge, ensuring the annotations remain relevant and accurate.
- Scalable Solutions: With its scalable annotation solutions, Remote Labeler can handle large volumes of data efficiently without compromising on quality or turnaround time.
Entrusting your microscopy data annotation to Remote Labeler not only resolves the challenges inherent in the process but also equips your AI models with the precision and accuracy necessary for revolutionary biomedical advancements.
Conclusion
The fusion of microscopy data annotation and artificial intelligence has been nothing short of transformational in the realm of biomedical image analysis. Data annotation, often an unsung hero, serves as the keystone in training AI models, empowering them to interpret biomedical images with an unrivaled level of precision and efficiency. Without this foundation, AI’s potential to bring a sea change in biomedical research and healthcare would be largely unfulfilled.
The world of healthcare and medicine is on the cusp of a revolution powered by AI, and the role of precise and reliable data annotation cannot be overstated. As we stand at the crossroads of this technological revolution, it becomes essential to harness the power of data annotation to its full potential. Remote Labeler, a trusted data annotation company, brings to you their expertise and resources to empower your AI capabilities. By ensuring top-quality annotations, Remote Labeler helps AI models to unlock the true potential of biomedical images, steering the way towards enhanced patient outcomes and groundbreaking medical research.
Empower your AI models with the precision of data annotation. Choose Remote Labeler for your data annotation needs today.
- Emerging Trends and Future Outlook: The Data Labeling Industry in 2024-2030 - December 8, 2023
- Landmark Annotation: Key Points - November 6, 2023
- All You Should Know About Bounding Box Annotation - November 5, 2023