In the realm of machine learning, the importance of a high-quality and properly annotated dataset cannot be overstated. A well-annotated dataset serves as the foundation for training accurate and reliable AI models. However, creating and labeling datasets can be a complex and time-consuming task. This article explores the solution of outsourcing dataset annotation, addressing the challenges associated with dataset creation and highlighting the benefits of leveraging professional annotation services provided by a trusted data labeling agency.
The Importance of a Dataset for Machine Learning
A high-quality, properly annotated dataset forms the bedrock of successful AI model training. It provides the necessary information and examples for algorithms to learn patterns, make predictions, and generate insights. Here’s why a dataset is crucial for machine learning:
Enhancing Model Performance
A well-annotated dataset empowers machine learning models to make accurate predictions and produce reliable results. Proper annotations, such as object bounding boxes, semantic segmentation masks, or text annotations, provide the ground truth for training algorithms. The more comprehensive and accurate the annotations, the better the model’s ability to generalize and handle real-world scenarios.
Avoiding Bias and Inaccuracies
Inadequate or biased datasets can severely hamper model performance. Without diverse and representative data, machine learning models may struggle to handle variations in inputs or exhibit biased behavior. A comprehensive dataset, carefully labeled with attention to potential biases, helps mitigate these issues and ensures fair and unbiased predictions.
Enabling Transfer Learning
Transfer learning, a technique where pre-trained models are used as a starting point for new tasks, relies on well-annotated datasets. By leveraging existing annotated datasets, developers can fine-tune models for specific tasks with limited labeled data. This process saves time and computational resources, accelerating the development of new AI applications.
Examples of Poor Dataset Quality
Insufficient dataset quality can lead to subpar model performance and inaccurate predictions. For instance, in object detection tasks, if the dataset lacks precise bounding box annotations or contains ambiguous labels, the resulting model may struggle to identify objects accurately. Similarly, in text annotation datasets, errors or inconsistencies in labeling can hinder natural language processing tasks. Poor dataset quality jeopardizes the reliability and usefulness of AI models.
The Challenges of Creating and Labeling a Dataset
Creating and labeling datasets pose several challenges, making it impractical for companies to handle this process in-house. Here are the key hurdles involved:
Complex Annotation Techniques
Dataset annotation requires expertise in various annotation techniques, such as object detection, semantic segmentation, or text labeling. These techniques demand a deep understanding of annotation tools, labeling guidelines, and domain-specific requirements. In-house teams may lack the specialized knowledge and resources to execute these complex annotation tasks effectively.
Time and Resource Constraints
The process of creating and labeling datasets demands significant time and resources. Companies need to allocate manpower, infrastructure, and annotation tools to carry out the task. These resource investments may divert attention from core business activities, causing delays in AI model development.
Scalability and Volume
For large-scale machine learning projects, handling dataset creation and annotation in-house can become overwhelming. Companies may encounter challenges in scaling annotation operations to accommodate the volume of data required. Recruiting, training, and managing a dedicated annotation team can prove costly and time-consuming.
Maintenance and Updates
Datasets often require periodic maintenance and updates to account for changing requirements, new data sources, or evolving annotation standards. Maintaining an in-house annotation team for long-term dataset management can be a significant challenge, especially when considering the need for continuous monitoring and quality control.
The Solution: Outsourcing Dataset Annotation
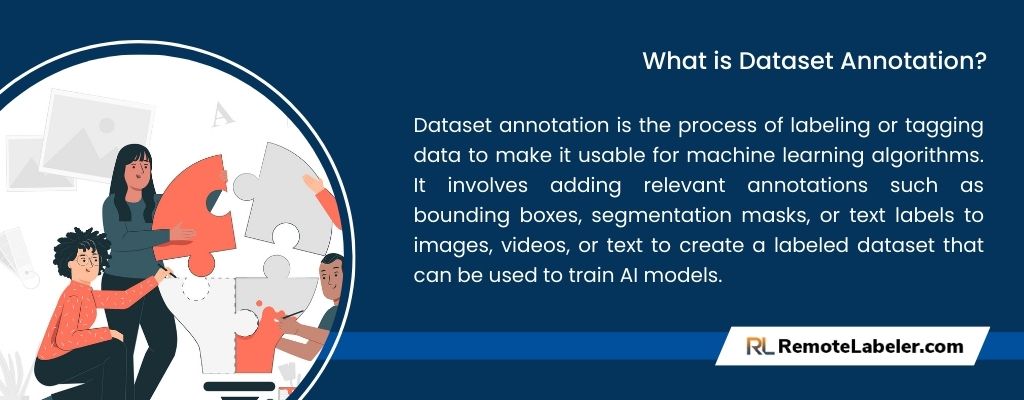
Outsourcing dataset annotation offers an efficient and effective solution to overcome the challenges associated with dataset creation and labeling. By partnering with professional annotation service providers, companies can leverage the following benefits:
Expert Annotation Skills: Dataset annotation experts possess the domain knowledge and technical proficiency required to execute complex annotation tasks accurately. Their experience ensures high-quality annotations that enhance model performance.
Scalability and Flexibility: Outsourcing allows businesses to scale annotation operations effortlessly, accommodating varying dataset sizes and project requirements. Service providers have the resources and workforce to handle large volumes of data efficiently.
Reduced Time and Costs: By outsourcing, companies save time and costs associated with recruiting, training, and managing an in-house annotation team. Moreover, professional annotation services optimize annotation workflows, ensuring timely project delivery.
Access to Advanced Annotation Techniques: Annotation service providers stay updated with the latest annotation methodologies, including popular formats like COCO dataset annotation and techniques like image dataset augmentation annotation. Leveraging their expertise ensures annotations adhere to industry standards.
Continuous Maintenance and Quality Control: Dataset annotation providers offer ongoing maintenance and quality control, ensuring datasets remain up-to-date and accurate. Regular audits and checks safeguard against errors, biases, and inconsistencies.
Dataset Annotation: The Solution to Your Dataset Woes
When faced with the challenge of not having the required dataset for machine learning, dataset annotation emerges as a powerful solution. Dataset annotation, including OCR annotation, involves the process of labeling and annotating data, providing the necessary information for training AI models. This section introduces dataset annotation as a solution and explains the process, including the tools and techniques employed.
Dataset Annotation Process
The dataset annotation process begins with understanding the specific requirements of the machine learning task at hand. Depending on the type of data, various annotation techniques are used. Common annotation types include:
Image Annotation: In image annotation, objects or regions of interest within an image are labeled with bounding boxes, polygons, or keypoints. This process enables machine learning models to identify and understand objects in images accurately.
Text Annotation: Text annotation involves labeling and categorizing text data, such as sentiment analysis, named entity recognition, or text classification. This annotation enables natural language processing models to analyze and interpret textual information.
Semantic Segmentation: Semantic segmentation annotates each pixel in an image with a specific class label, allowing machine learning models to understand the structure and context of objects within the image.
To execute the annotation process, annotation tools and software are used. These tools enable annotation experts to label data accurately and efficiently, ensuring high-quality annotations for training AI models.
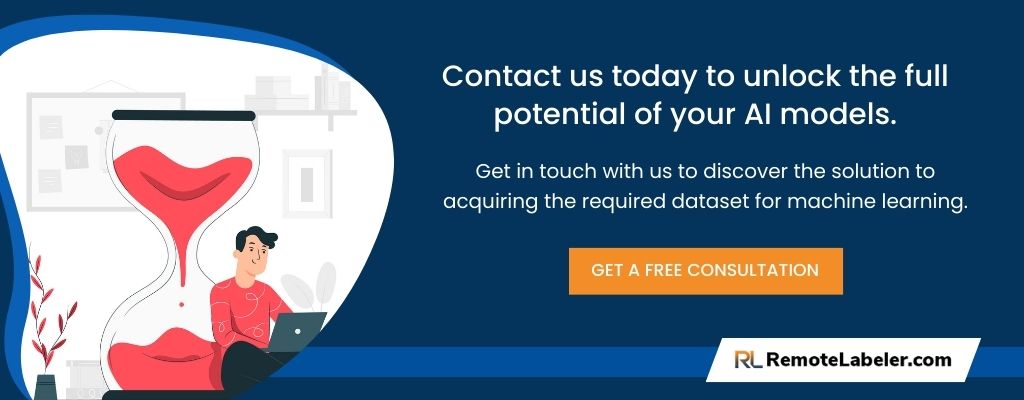
The Benefits of Outsourcing Dataset Annotation
Outsourcing dataset annotation offers numerous benefits to companies that lack the required datasets for machine learning. Let’s explore some of these advantages:
Cost-Effectiveness
Outsourcing dataset annotation is a cost-effective solution for companies. Establishing an in-house annotation team requires substantial investments in recruitment, training, and infrastructure. By outsourcing, businesses can access professional annotation services without the need for extensive upfront costs, optimizing their budget allocation.
Access to Expertise
Dataset annotation service providers specialize in the annotation process and employ experienced annotation experts. These professionals possess domain knowledge and expertise in diverse annotation techniques, ensuring accurate and reliable annotations. Leveraging their skills and knowledge enhances the quality and effectiveness of the annotated dataset.
Scalability and Flexibility
Outsourcing dataset annotation offers scalability and flexibility. Dataset annotation service providers have the resources and capacity to handle datasets of varying sizes and complexities. They can adapt to fluctuating annotation requirements, accommodating large-scale projects or datasets with ease. This scalability ensures timely delivery and reduces bottlenecks in AI model development.
Case Studies or Examples
To illustrate the benefits of outsourcing dataset annotation, let’s consider a case study. Company X, a startup in the healthcare industry, required a large annotated dataset for training a deep learning model to detect anomalies in medical images. However, they lacked the resources and expertise to annotate the dataset in-house.
By outsourcing dataset annotation to a specialized data labeling agency, Company X gained access to a team of experienced annotators who employed advanced annotation techniques. This enabled them to annotate a significant volume of medical images accurately and within the required timeframe. The annotated dataset played a crucial role in training a highly accurate AI model, providing valuable insights for medical diagnosis.
Choosing the Right Service Provider for Outsourcing Dataset Annotation
When outsourcing dataset annotation, it is essential to choose the right service provider. Here are some factors to consider:
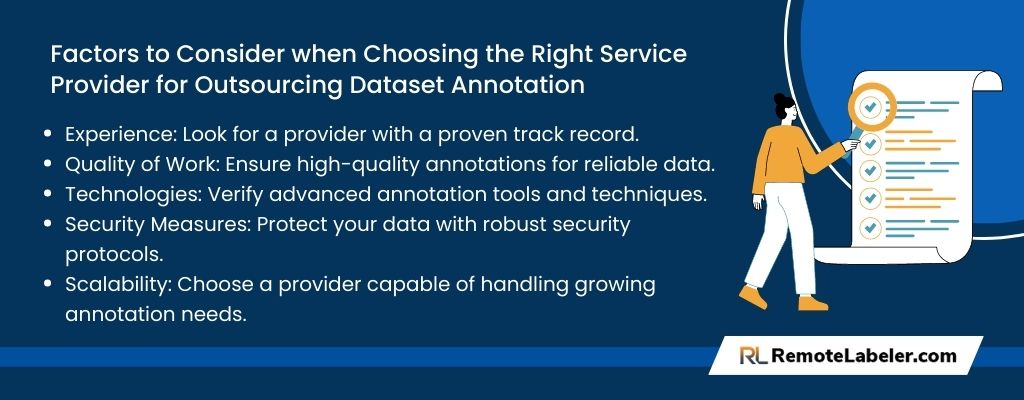
Experience: Look for a dataset annotation service provider with a proven track record and experience in the field. Experience ensures expertise in annotation techniques and the ability to handle diverse datasets.
Quality of Work: Quality is paramount when it comes to dataset annotation. Assess the service provider’s quality assurance processes, including multiple rounds of review and validation, to ensure accurate and reliable annotations.
Technologies and Tools: Ensure that the service provider utilizes advanced annotation tools and technologies. This includes support for industry-standard formats like COCO dataset annotation format and proficiency in image dataset augmentation annotation techniques.
Security Measures: Data security is critical when outsourcing dataset annotation. Evaluate the service provider’s security measures, such as encryption, restricted access, and confidentiality agreements, to ensure the protection of your data.
Considering these factors will help you select a dataset annotation service provider that meets your specific requirements.
To address your dataset annotation needs, we invite you to reach out to Remote Labeler, a leading data labeling agency. With extensive expertise, advanced annotation techniques, and a commitment to quality, Remote Labeler can assist you in creating high-quality datasets for your machine learning projects.
Conclusion
In conclusion, lacking a required dataset for machine learning can pose significant challenges. However, outsourcing dataset annotation provides a practical and effective solution. By leveraging professional annotation services, companies can overcome the complexities and resource constraints associated with dataset creation and labeling.
Outsourcing dataset annotation offers numerous benefits, including cost-effectiveness, access to expertise, scalability, and flexibility. Case studies exemplify the advantages of outsourcing, showcasing successful applications of annotated datasets in training AI models.
When choosing a service provider, consider factors such as experience, quality of work, technologies, and security measures. Remote Labeler stands as a trusted dataset annotation service provider, equipped with the expertise, experience, and commitment to quality necessary to meet your annotation needs. Take advantage of the benefits of outsourcing dataset annotation and unlock the full potential of your machine learning projects.
- Emerging Trends and Future Outlook: The Data Labeling Industry in 2024-2030 - December 8, 2023
- Landmark Annotation: Key Points - November 6, 2023
- All You Should Know About Bounding Box Annotation - November 5, 2023