In the rapidly evolving landscape of autonomous vehicles, the role of data collection has become paramount in propelling the development of artificial intelligence (AI) algorithms. As self-driving cars seek to revolutionize transportation with their potential for enhanced safety and efficiency, the process of data annotation takes center stage. Data annotation service providers play a pivotal role in this domain, facilitating the collection and labeling of vast and diverse datasets that serve as the foundation for training AI algorithms. In this article, we explore the significance of data collection for AI development in the context of autonomous vehicles, shedding light on the various types of data annotation involved, and highlighting the remarkable advantages of AI-driven self-driving cars.
Autonomous vehicles, also known as self-driving cars, hold the promise of reshaping the future of transportation with their potential to navigate roads without human intervention. These cutting-edge vehicles rely on a sophisticated network of sensors, including LiDAR, cameras, radar, and GPS, to perceive their surroundings and make informed decisions in real-time. However, the seamless functioning of AI algorithms powering self-driving cars heavily relies on the data they are trained upon. This is where data annotation service providers play a critical role, facilitating the collection, labeling, and annotation of diverse datasets to enable AI algorithms to recognize road conditions, interpret traffic scenarios, and ensure safe and efficient navigation. By delving into the world of autonomous vehicle data collection, we can better understand how these modern marvels are empowered to embrace the road ahead with intelligence and innovation.
Introduction to Self-Driving Cars and AI Development
Self-driving cars, a culmination of cutting-edge technologies, artificial intelligence, and automotive innovation, are vehicles equipped with the ability to navigate and operate without human intervention. These autonomous vehicles leverage a combination of advanced sensors, powerful onboard computers, and sophisticated AI algorithms to interpret and respond to their surroundings in real-time. The integration of LiDAR, cameras, radar, and GPS enables self-driving cars to gather vast amounts of data about the environment, effectively creating a digital representation of the world around them.
Key Components of Self-Driving Cars:
- LiDAR (Light Detection and Ranging): LiDAR technology utilizes laser beams to create high-resolution 3D maps of the vehicle’s surroundings, measuring distances and accurately identifying objects.
- Cameras: Cameras capture real-time images and videos, providing crucial visual data for object detection, lane tracking, and traffic sign recognition.
- Radar: Radar sensors use radio waves to detect the distance, speed, and direction of objects, enhancing the vehicle’s ability to perceive its environment, especially in adverse weather conditions.
- GPS (Global Positioning System): GPS receivers provide precise location information, aiding in navigation and route planning.
The AI development for self-driving cars revolves around creating complex algorithms that process the data collected from various sensors. Machine learning techniques, including deep learning and neural networks, enable the AI models to learn from the data and make intelligent decisions. Through continuous exposure to diverse datasets, these algorithms can adapt and improve their performance, refining their ability to accurately interpret traffic scenarios, identify obstacles, and navigate through intricate road systems. The combination of advanced AI and sensor technologies enables self-driving cars to not only follow predefined routes but also respond to unpredictable and dynamic road conditions, making them safer and more efficient than traditional vehicles.
The Importance of Autonomous Vehicle Data Collection
Before autonomous vehicles can confidently navigate the roads, they must undergo rigorous training through massive datasets that capture real-world driving scenarios. The process of autonomous vehicle data collection serves as the lifeblood of AI development, providing the crucial information needed to train and fine-tune AI algorithms. These datasets encompass a wide array of road conditions, traffic patterns, weather variations, and unforeseen events, enabling self-driving cars to adapt swiftly and make informed decisions in complex driving environments. The richness and diversity of the collected data lay the groundwork for safer, more efficient, and reliable autonomous driving experiences.
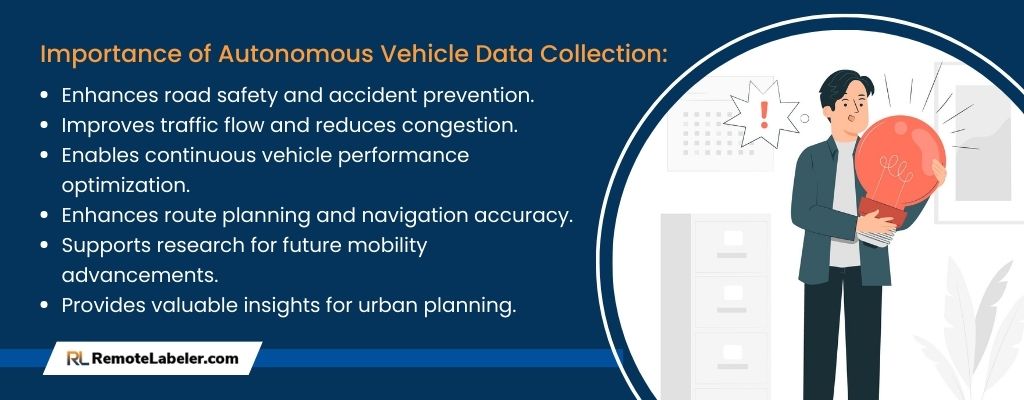
Key Aspects of Autonomous Vehicle Data Collection:
- Vast and Diverse Data: The collection of extensive and diverse datasets is essential for developing AI algorithms that can handle a wide range of driving scenarios. Real-world data reflecting diverse conditions enhances the AI’s ability to identify and respond to various traffic situations with accuracy.
- Real-Time Adaptability: Autonomous vehicles must be equipped to respond promptly to rapidly changing traffic conditions and unexpected events. Continuous data collection and real-time processing enable AI algorithms to adapt on-the-fly, ensuring a smooth and safe driving experience for passengers and pedestrians alike.
- Uncommon Scenarios: Autonomous vehicle data collection goes beyond routine driving situations to include rare and critical events. By exposing AI algorithms to uncommon scenarios, such as accidents or extreme weather conditions, self-driving cars can learn to react appropriately and mitigate potential risks.
- Quality and Precision: The quality and precision of the collected data significantly impact the performance of AI-driven self-driving cars. Data annotation service providers play a crucial role in ensuring accurate and meticulous labeling, providing the necessary context for AI algorithms to interpret the environment effectively.
- Iterative Learning: Data collection is not a one-time process; it is an iterative learning cycle. As self-driving cars traverse roads, they continuously collect and analyze new data, refining their AI models to enhance driving capabilities and overall safety.
In the journey towards fully autonomous vehicles, the importance of data collection cannot be overstated. The wealth of data obtained and the precision in which it is annotated shape the intelligence and responsiveness of AI algorithms, empowering self-driving cars to navigate the roads with confidence and ushering in a new era of smart and efficient transportation.
Data Annotation Types for Autonomous Vehicles
To train AI algorithms effectively for autonomous vehicles, the process of data annotation involves various techniques that encompass different aspects of the vehicle’s environment. Each annotation type contributes to enhancing the AI model’s perception and decision-making capabilities, making it critical for achieving reliable and safe autonomous driving. Let’s explore the key data annotation types used in autonomous vehicle development:
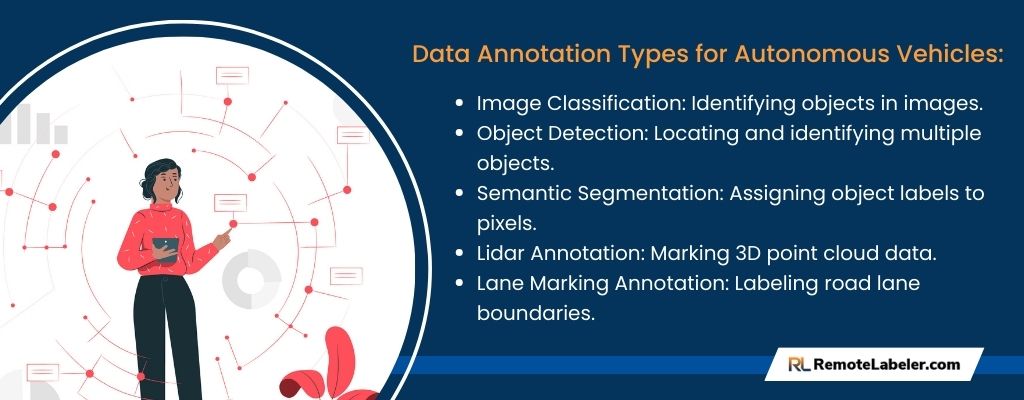
1. Image Annotation
Image annotation involves the meticulous labeling of objects, pedestrians, traffic signs, lane markings, and other relevant entities present in camera images captured by the autonomous vehicle. This data is essential for teaching the AI system to recognize and respond to the diverse elements that exist in the surrounding environment, enabling it to make informed decisions while navigating through complex traffic scenarios.
2. LiDAR Point Cloud Annotation
LiDAR technology generates 3D point cloud data, representing the distance and shape of objects surrounding the autonomous vehicle. Annotating LiDAR point clouds plays a crucial role in accurate object detection, collision avoidance, and understanding the precise spatial relationships between various elements on the road.
3. Semantic Segmentation
Semantic segmentation provides pixel-level annotation, allowing the AI model to comprehend the exact boundaries and semantic meaning of different objects on the road. This fine-grained annotation technique is vital for the autonomous vehicle’s precise navigation and decision-making, as it helps differentiate between various elements present in the environment.
4. Instance Segmentation
Instance segmentation takes semantic segmentation a step further by providing pixel-level annotations for individual instances of objects. This means that the AI system can distinguish between multiple instances of the same object class, such as different vehicles or pedestrians. Instance segmentation enables the vehicle to respond more accurately to the presence of specific objects and enhances its ability to adapt to complex scenarios on the road.
By leveraging a combination of these data annotation types, autonomous vehicle developers can create robust and intelligent AI models capable of comprehending their surroundings and making safe decisions in real-world driving situations. The precision and accuracy of data annotation significantly impact the performance and reliability of autonomous vehicles, driving us closer to a future where self-driving cars are an integral part of our daily lives.
How We Can Help: Remote Labeler Expertise
At Remote Labeler, we take pride in being a leading data annotation service provider with extensive expertise in the field of autonomous vehicle data annotation, as well as outsourced retail data annotation. Our specialized team of professionals is well-versed in the complexities of annotating diverse datasets required for training AI algorithms in self-driving cars. We understand the critical role that high-quality data plays in the development of autonomous vehicles and retail applications alike, and our commitment to excellence ensures that our clients receive the best-in-class annotations for their AI-driven projects.
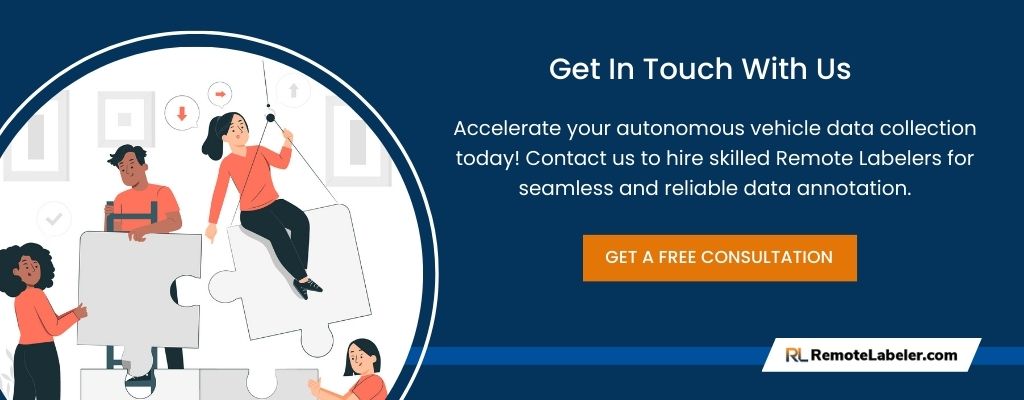
1. Domain Knowledge
Our team possesses in-depth domain knowledge, allowing us to understand the specific requirements of autonomous vehicle data annotation. We are well-acquainted with the challenges and intricacies of training AI models for self-driving cars, enabling us to provide precise and accurate annotations that align with the objectives of the project.
2. Cutting-Edge Tools and Technology
To maintain the highest standards of data annotation, we employ cutting-edge annotation tools and technologies. From image annotation to LiDAR point cloud annotation, our advanced tools streamline the annotation process, ensuring efficiency without compromising on quality.
3. Customized Annotation Solutions
We recognize that every autonomous vehicle project is unique, and data annotation requirements can vary significantly. At Remote Labeler, we offer customized annotation solutions tailored to meet the specific needs of each project. Whether it’s semantic segmentation, instance segmentation, or a combination of techniques, we have the flexibility and expertise to deliver the desired results.
4. Quality Assurance
Quality assurance is at the core of our data annotation process. We have strict quality control measures in place to ensure that every annotation meets the highest standards of accuracy and consistency. Our rigorous quality checks ensure that the AI models trained on our annotated datasets perform optimally, leading to safer and more reliable self-driving cars.
5. Scalability and Timeliness
We understand the importance of scalability and timeliness in autonomous vehicle development. With the capability to handle large-scale datasets efficiently, we can accommodate projects of varying sizes while adhering to strict timelines. Our focus on delivering results promptly enables our clients to accelerate their AI development and deployment processes.
Conclusion
In the quest to empower self-driving cars and advance AI development, data annotation is a crucial enabler that lays the foundation for reliable and intelligent autonomous vehicles. At Remote Labeler, we stand committed to providing top-notch data annotation solutions for autonomous vehicles, harnessing our expertise and cutting-edge technology to drive innovation in the automotive industry. As the industry transitions toward a future where self-driving cars become a reality, our data annotation services play an integral role in shaping a safer, more efficient, and sustainable transportation landscape. Let Remote Labeler be your trusted partner in the journey of creating AI-driven autonomous vehicles that revolutionize the way we move and transform the world of transportation.
Unlock the Power of Remote Labelers Today! Hire Skilled Remote Labelers for Precise and Efficient Data Annotation.
- Emerging Trends and Future Outlook: The Data Labeling Industry in 2024-2030 - December 8, 2023
- Landmark Annotation: Key Points - November 6, 2023
- All You Should Know About Bounding Box Annotation - November 5, 2023